The new year kicks off with two paper acceptances. “Auditory model based subsetting of head-related transfer function datasets” (S. Spagnol) will be presented at the 45th IEEE International Conference on Acoustics, Speech, and Signal Processing (IEEE ICASSP 2020) taking place in Barcelona, Spain in May. We will also be in Atlanta, US next month at the IEEE 5th VR Workshop on Sonic Interactions in Virtual Environments with the paper “HRTF individualization using deep learning” (R. Miccini, S. Spagnol), as part of the 27th IEEE Conference on Virtual Reality and 3D User Interfaces (IEEE VR 2020).
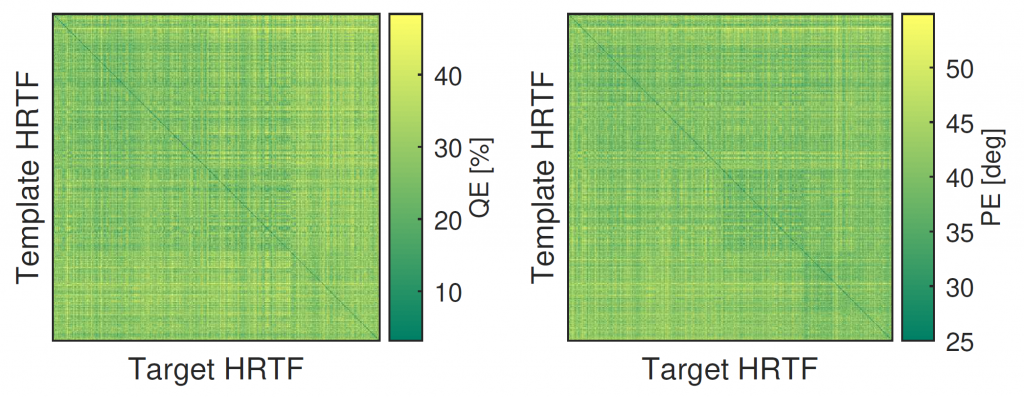
The former paper outlines a novel HRTF subset selection algorithm based on auditory-model vertical localization predictions and a greedy heuristic to identify an optimal subset of representative HRTFs from a catalogue including the three biggest open HRTF datasets currently available online (see figure above). An objective validation of the optimal subset on a fourth independent dataset, based again on auditory model predictions, is provided. The results show an overwhelming agreement on the choice of this optimal subset, supporting the idea that a large HRTF catalogue can be efficiently reduced by two orders of magnitude while preserving at least one HRTF fitting the very large majority of a pool of listeners in terms of localization error.
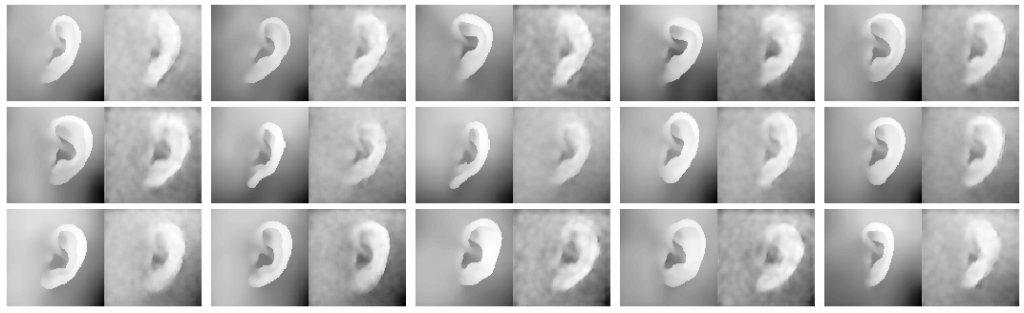
The research presented in the latter paper focuses on HRTF individualization using deep learning techniques. The rising availability of public HRTF data currently allows experimentation with different input data formats and various computational models. Accordingly, three research directions are investigated: (1) extraction of predictors from user data (see figure above) ; (2) unsupervised learning of HRTFs based on autoencoder networks; and (3) synthesis of HRTFs from anthropometric data using deep multilayer perceptrons. While none of the aforementioned investigations has shown outstanding results to date, the knowledge acquired throughout the development and troubleshooting phases highlights areas of improvement which are expected to pave the way to more accurate models for HRTF individualization.